
Here is an interesting conundrum from Lipton (2005).
Let’s call it the “Twin Scientist Problem.”
The Twin Scientists
Once upon a time there were identical twin scientists. They looked so similar it was hard to tell them apart. One day you run into them at a conference, where they are standing next to their respective posters.
You read over both posters. They make the exact same claims and are supported by the exact same data. In fact, they are like identical twin posters…except for one, small thing.
Twin A collected his data AFTER formulating his hypothesis. He generated a hypothesis and then collected his data to test it.
Twin B collected his data BEFORE formulating his hypothesis. He collected his data and then generated a hypothesis to explain it.
Still, the twins have the same hypotheses, the same datasets, and are claiming the data support the same results.
But they’re not the same.
Twin A’s claim is stronger than B’s.
How can this be?

The answer has to do with the concept of “confounds.”
First, here’s an interesting twist.
As Lipton asks, if Twins A and B didn’t know about each other and met for the first time at the conference, what should THEIR reactions be to seeing each other’s research?

Twin B should increase his confidence but Twin A should not. Twin B had no way of knowing whether his data contained confounds and little reason to assume they didn’t.
What Are Confounds?
A “confound” is a variable OTHER THAN THE ONE HYPOTHESIZED that might be the real explanation for the pattern observed in the data. If you did not account for it, if you did not design the research to control for it, then making causal claims will be problematic.
It’s easier to illustrate with examples. So here are some claims….
Example 1:
Children who sleep with their lights on at night are more likely to become nearsighted later in life.
Example 2:
Drinking water contaminated with asbestos can cause lung cancer.
Example 3:
Moderate drinkers are healthier than teetotalers, so moderate drinking must have health benefits.

These are all real claims that have been made by real researchers. Can you think of the confound present in each?
In the first example, nearsighted parents are more likely to have nearsighted kids, period. They are also more likely to leave the lights on at night BECAUSE they are nearsighted. Genetics is the causal variable, not leaving the lights on.
In the second example, an older one, poverty is likely the confound. It’s a third variable correlated with both the observed variables in the study (asbestos and cancer). (Poor people are also far more likely to smoke.) A similar example common in stats classes is that “ice cream causes crime.” (Heat causes increases in both.)

The third example has been all over the news lately. Research comparing drinkers to teetotalers is confounded by people who CANNOT drink because of existing health problems. Controlling for this, what the research is now showing is that no amount of drinking is ever healthy.

Prediction vs. Accommodation
Where does this leave us? Well, because of confounds, predicting effects is considered more scientific than explaining them after the fact.
Lipton gives a fascinating example of why this is the case. Say data come in that conflict with your view that the planets have circular orbits. You then posit the existence of “epicycles” to make your model gel with the new data.
Notice the data do not test your new epicycle hypothesis; rather, your hypothesis is an attempt to EXPLAIN AWAY the new data. This is a crucial difference, and is why some scientists pejoratively refer to ad hoc hypothesizing as “adding epicycles.”
Remember from above, Twin A formulated a hypothesis and then collected data to TEST it. Twin B collected data and then formulated a hypothesis to EXPLAIN the results. In other words, Twin A’s hypothesis was a prediction while B’s was an accommodation.
Twin A designed an experiment as a test his prediction could fail, allowing him to control for confounds. Twin B collected his data and then tailored a hypothesis to it. That’s fine for GENERATING hypotheses, just not for TESTING them. In fact, Twin B’s work could have provided the hypothesis and then Twin A’s could have tested it.
In general, you should not use the same data both to generate and test the same hypothesis. If data are used to generate a hypothesis, other observations must be made, and other data collected, to then test it.
To close, it doesn’t really matter that the data and the wording of the “twin” hypotheses are the same. The RELATIONSHIPS between the data and hypotheses are what matters.
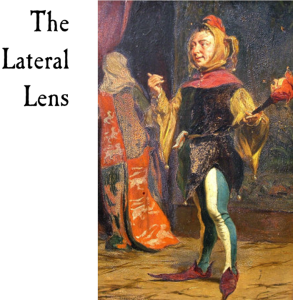
Reference
Lipton, P. (2005). Testing hypotheses: Prediction and prejudice. Science, Vol. 307, 219–221.
One thought on “Lipton’s Twin Scientist Problem”